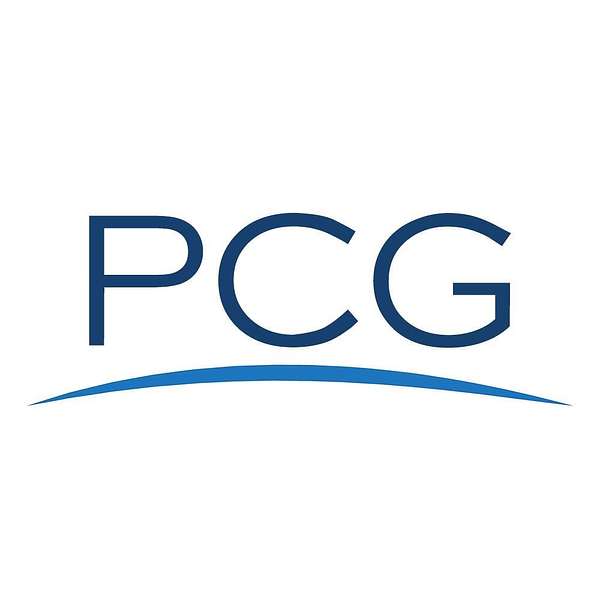
Panorama Consulting Group - The Panorama Angle
Panorama Consulting Group - The Panorama Angle
Machine Learning in Manufacturing: 5 Tips for Leveraging Data Insights
Machine learning and AI are more accessible than you might think! With the right approach, manufacturing companies of any size can quickly gain value from these technologies. The key is understanding how to generate data insights that result in both strategic and tactical benefits.
In this podcast episode, Paul Dunn, VP of Product Management at Infor, shares five tips for gaining valuable data insights from machine learning and AI. Be sure to tune in to this episode before implementing any of these advanced (yet accessible) technologies.
Welcome
Speaker 2:To the Panorama angle podcast, where we provide expert advice on everything from E R P selection to business transformation. Today, I'm pleased to welcome Paul Dunn VP of product management at N four. Today we will be discussing five tips for leveraging machine learning. Data insights in four is a provider of business cloud software products for companies and industry specific markets Infor builds complete industry suites in the cloud and deploys technology that puts the user experience. First leverages data science and integrates easily into existing systems. With that. Let's go ahead and dive deep into machine learning. Paul, tell me a little bit more about the role of machine learning and manufacturing.
Speaker 3:Well, first of all, thank you for having me here on the podcast. And, and I think one of the things as we start off manufacturers are really struggling to deal with volatility in the supply chain right now, um, dealing with business and manufac anomalies, predictive quality control and, and maintaining their products so that they're getting a very good product or knowing when they're going to produce a good product capturing their internal knowledge. And, you know, one of the more typical use cases that manufacturers are using machine learning for is predictive and preventive maintenance. Those things are the things or the areas of focus that many manufacturing companies are, are trying to struggle with right now. Um, many of the larger companies, if you will have been able to go ahead and spend money to do that because they can afford to do that. But I think many small and medium size manufacturers, uh, maybe have struggled to leverage all of the data and insights they have at their fingertips. Um, perhaps they're also getting caught up in a little bit of emphasizing a little too much of the sizzle out there versus really looking at what's the stake out there. What are the things that they can focus on getting real value out of? And so really those are some of the things I think we'd like to talk about today.
Speaker 2:OK. So artificial intelligence and machine learning have been portrayed both in the industry and, uh, via entertainment, especially. So like within movies in particular like drawn Terminator, that kind of thing, and are shown as being very advanced in their capability. So what's a more realistic view of where businesses can expect value from AI ML systems.
Speaker 3:So a realistic view of what business businesses can expect from AI and ML. Let's, let's step back a little bit Hollywood, and to some extent, larger companies and maybe some of the product suppliers have really kind of moved AI into this realm where we almost feel like some of the capabilities are fantasy. Uh, you know, many of our suppliers, they can supply systems that do things that are really amazing, but it's kind of complicated to figure out what's real and what's fiction in the marketplace. And a great example. If you look at some of the things you see in the movies like, uh, we see, um, unfortunately in things like Terminator and iRobot and things like that, these really sophisticated intelligences that are out there. And, and that's not really the reality of what artificial intelligence and machine learning and where we're at as a, a, as an industry right now, I think we've focused a little bit too much on highly complex and functions. Um, and, and to get that really, uh, highly complex feature and function tool set, it's really expensive and complex. And I would say that many of our mid and small size businesses, uh, really can't pay for even, uh, a fraction of that capability. It, it, it become an issue of, am I getting value from what I'm paying for? Um, I think companies in this marketplace, many companies would be much better served if they, they tried to go for a good enough answer that leads to real value creation for them. So when we think about that, I, um, thinking about value and how you created, uh, the results, how those results from an intelligent model are in understood disseminated, and action is triggered as a result and, and you don't need to be complex, but the models do need to be able to with adapting variables. And a great example of that is COVID. For example, if you think about demand forecasting, my goodness, what a huge change the COVID pandemic was rapidly in the marketplace, you have to be able to adapt, uh, to some, an unusual like that within a reasonable amount of time companies that start with, I think, simple processes and analyses, and then they branch out based on those learnings will probably see the most benefit from artificial intelligence and machine learning. Um, rather than trying to start really complex and start with the big bang, if you will. I think it's, it's probably a little counterintuitive start, start simple and build upward rather than start complex and build downward.
Speaker 2:Okay. That's some great, uh, good general knowledge there and a really great overview. Uh, so diving a little bit more specific, are there specific areas of intelligence where customers can apply intelligent models?
Speaker 3:Yeah. Um, from the standpoint of major areas of focus, I think if, if you're trying to find some returns on value, there are six that, that we kind of an Infor kind of divide artificial intelligence and machine learning into, um, because they're simple to explain. And, and the first of those is process intelligence. So improving your efficiency and decision making for both business and manufacturing processes. So that can be as simple as how do I automatically detect anomalies in my accounting system to prevent fraud. Okay. That is something that is really a simple thing for artificial intelligence and machine learning, to capture. And it's part of my business process in a manufacturing side. It, my might be something as simple as a manufacturer trying to determine what is my optimal settings of my manufacturing process or the optimal, um, ingredients in a recipe to get me a perfect product every time to know those things. And so those are really good areas with within the process intelligence segment, uh, that companies can invest in and see really concrete results. Asset intelligence is the second one, and that's the one that's probably the most, um, known by the business community. So when we talk about asset intelligence, we're talking about predictive and preventive maintenance, and being able to predict when a piece of equipment might, uh, not work as it's supposed to, or have unexpected downtime, which is really one of the leading causes of, uh, lost opportunity and lost value for companies when they're in production. And, uh, a piece of equipment suddenly will go down for maybe day, days, or weeks at a time. So that's one that's really well, a well known and well documented. It's probably the most populous of all of the AI ML engines that are out there. Forecasting intelligence is one of the big ones that's really coming into. Um, it's, it's more necessary for companies to have, and that's because of supply chain issues, right? How, how am I going to react, um, to, within my supply chain. Um, and, and when should I know when to order material, et cetera. And now that we have these, these variabilities in the supply chain, knowing what I need to know, uh, knowing what I need to buy and when to have it become really, really important, and even predicting when some of that material might be shorted, wow, that becomes a really big, uh, impact to the bottom line. For many customers, sales intelligence, their customer sales intelligence is the fourth one where you can think about how do I improve my efficiency and effectiveness of my customer relationships, who are my best potential target customers. All right. How do I really forecast, um, my sales goals for the coming year and boy having, having been through that process many times, it seems like a black art. Uh, at times there are ways to make that a little bit more accurate. The fifth one is pricing intelligence. How do I drive market centric pricing and detect pricing anomalies or opportunities in the market, uh, where I might be able to raise my price and increase my margins, as well as where might my margins be shrinking and how might I deal with that is another area, uh, to work in, uh, in the IML sector. And finally, one that I think is just emerging and that's human capital intelligence, where you really can improve your labor efficiency, um, improve your employee satisfaction and retention. I mean, absolutely being able to identify client or not clients, but, um, employees who might be at risk before you lose them. And, and with the tight labor markets that we have, even now, that's an important lesson. You don't wanna have your talent walking out the door and the knowledge that you have walking out the door. Um, it'd be great. If you could know when potentially your, your, your employees are unhappy in their situation. And there are ways to detect that as well. In addition with the labor force, with the graying of the labor force, as we continue to see that there's also a really great way of gaining some of the, that those employees have and building it back into your processes. So those are the areas I would suggest that many manufacturers should really look in. There's lots of use cases out there for them. If you, you scour the internet, you can find, uh, many of them, but starting those would be great areas for most companies to really investigate and see where they could find some value.
Speaker 2:Yeah, you're definitely right. There are obviously a lot of use cases and ways that companies can kind of go start their journey with AI and ML solutions. Uh, I feel like it can be pretty intimidating on how to begin though. So how would, uh, how would I know whether I need new sensors or real time systems with the help of intelligence to make decisions?
Speaker 3:Yeah. And this is actually one of the bigger questions that we keep getting asked is don't, I need to make this great investment in new information. I O T systems, uh, don't. I need to bring all of my day into a data lake don't. I have to organize all of this data, and I think the answer's are qualified maybe. And I think this is kind of the second tip that I have for folks on this call or on this, uh, podcast, is that if, if we have data and, and we do have data, most, most enterprises do have significant amounts of data, either within their E R P systems, their CRM systems, HR, um, maintenance management systems, uh, manufacturing, execution systems, as well as believe it or not Excel spreadsheets, um, and sometimes just digitized pieces of paper. Um, there's a lot of information that companies can already do a great deal of work with. And this really is dictated by a simple question. So you keep hearing about IOT and all of these sensors, and, oh, let's go out and buy stuff. But there's one simple question that really kind of tells you whether you need to invest in that. And that's the speed that you require to react to changing information. So, great example of this, as you, as you have more strategic information. Um, so in, for example, a predictive maintenance application, most of the time, most AI and ML within that space cannot really tell within an hour that that machine is going to break. They actually take a little bit more time, but by the time you have that you have enough information, um, it's already starting to fail. The idea is to actually widen that window as much as possible, and you don't need as much detailed information as you think you do in order to put a good prediction in place to know when that asset's going to fail. So I actually need less information if I wanna narrow that time window or be explicitly accurate. Okay. Let's say I'm three months away from a potential maintenance. And I say, oh, okay. It is 90% likely that in six months that machine's gonna fail. I'm in good shape. If I wanna say, oh, I'm that machine's going to fail next week. I do need to have much more information to understand why it's going to fail in one week versus three months or six months out, which seems kind of strange, but it's, it's a very inverse relationship. So most companies are sitting on a treasure trove of information. If I need to do strategic planning, that's typically a slower burn than something that's very tactical. So I would say the more that you, you have a strategic situation, the more the data you already have is probably very good for your needs, right? If you want to have an extremely tactical situation where I needed to make quick decisions, that's when you need to bring in these IOT sensors and new informations, new information sources, so that you can get that granularity that you need to make those decisions. The problem with time, time to value for the latter type of projects where you're using lots of I O T sensors, and lots of information is that the time to value increases greatly, um, as you add all of that information, because you've got to put in the proper system to control that data, to organize that data and align it with each other, um, as you do AI and ML improvements that are more strategic in nature, because there's actually less data types. You can put those together much more quickly. So the time to value is higher. So I would say many of those types of strategic projects, you can actually accomplish them in weeks rather than months. So I've done projects where we're working with 10 or 10 to 30 variables, for example, and that's an eight week process, right? I've done projects where we're dealing with 2,500 variables, and that took six months to get prepped before we could actually do the work behind the artificial intelligence. Now, the funny part about that is actually once we got the data organized, it only took three weeks to discover the root causes, right. But it's that it's at putting everything together that actually takes a great deal of the work to get the value out of these types of projects. So keeping it simple and strategic is probably the best way to go for most companies.
Speaker 2:Okay. So with all that data, I mean, organizing and managing, that seems like a pretty be daunting challenge. Uh, so how would you go on about minimizing the amount of effort required to source the data to, to the models? Really?
Speaker 3:Yeah, this really comes down. Um, and I think this is one of the more challenging things for people to wrap their head around. So I'll try and explain this, um, in a, a, in a somewhat simplistic way, and it's, it's probably not this simple, but it's a good way of thinking about it. If you think about what information that I need to deliver to a certain type of result and build a data model that says, I need these types of information, that's called creating a semantic model. Okay. And it doesn't matter where that data exists from. As long as I pull the data that fits my model, and I can repeat that over and over again, it really makes it much easier to put it, put it together and get to an answer than what a lot of people are, are, have, have tried to do, which is let me all of the data that I have and mash it into a data lake. Okay. And then I'm gonna point to the data lake, and I'm gonna hope to get some results out of it. Okay. What ends up happening is you can end up with a lot of false correlation.<affirmative> when you're trying to do that, um, or you'll get results that might not make a lot of sense. So if, if you're able to parse, parse your systems a little bit and put a little planning into the data models behind, um, the AI and ML, um, and understand how those work, you can cut down on the amount of clutter that you bring into the process. So I, I would say it doesn't eliminate the need to organize your data, but it certainly can reduce the effort. And you can be very specific about the types of things that you're trying to do. Um, I have seen, and, and I know some of the folks that I work with on a regular basis, and you'll see this, uh, very widely spread now in industry, as people develop data models, they'll come with really great instructions about what types of data you need, what types of data you don't need for specific models to work, work for you. And what's really interesting about this concept is if you create a data model to do a specific task, let's say anomaly detection, okay. It really doesn't matter the type of anomal that I'm looking for. I can get the right type of data into that, and it will catch the anomaly, whether it's a video anomaly that it's getting from video or an accounting anomaly that it's getting from a, an E R P system, they really kind of function in very similar ways, deep down. So you can get a lot of use out of the models and then point the specific data that you want the model to process and come up with a result. Hopefully that's, uh, uh, kind of a simple way of explaining it. But I, I would say coming at the models with an idea in my, of what you're trying to do with them is, is probably the way to reduce the complexity of, uh, and, and the challenge of bringing all the data together.
Speaker 2:Okay. That's some really great information. Just a little bit of prep work can really reduce your overall effort, uh, that you kind of put into it. So once companies kind of start having success in doing this, what should be the driving or motivating factor to add more intelligence, uh, capacity or capability.
Speaker 3:So I think this goes back to part of what I was saying before about, um, what do I get by bringing more data and, and more complexity into my models. Okay. So let's just say that you have, uh, a forecasting model that you determine is about 85% accurate. That's pretty good and, and better than most companies can do. Now. I actually was on a phone call, uh, the other day, and I, I was talking to a client and that client, I asked them the question, well, how are you forecasting right now? And, and the client answered, well, we kinda wet our fingers, stick it up in the, the air and kind of figure out which way the wind's blowing. Well, I can tell you 85% accuracy on a data model, even if it's simple. One is certainly better than that. Uh, and certainly brings more certainty to that client, um, as they're going forward, but there's always the folks that are gonna wanna try and push that envelope further. Right. Um, and that let's say I want to go from 85% com uh, uh, accuracy to 90 and 95% accuracy, which would be a real trick, to be honest with you in the demand forecasting realm. So adding complexity and capability and speed is great. Once you have achieved a certain level of success, I would recommend you start, you know, at the simple end, and you can talk about adding real time data such as those supplied by automation systems. I O T devices from the internet from a variety of sources and, and thinking about data that can come from many different places that will increase the accuracy of your models as your time window reduces, as we talked about earlier. So I can give you a couple examples of that, um, identification of a specific type of failure. Um, as we, as we gain more process variables, uh, from the system, if we add video, maybe audio is being experimented with folks, you can bring all of this complicated in information in to identify a specific type of failure where before I would just be able to, I'm probably gonna have a failure here, but I don't necessarily know what part or what component, um, within a, a piece of equipment might fail. If I want to have dynamic optimization of my production based on my, my current machine status. Um, being able to grab all of that data from all of these different machines, um, and bringing it together and working it over, over again, and looking at scenarios to it becomes a very complicated effort, right? The intelligence behind it is not as difficult, but you're repeating that process over and over again. And that gets very expensive. If you wanna look at individual impacts by an employee upon production, okay, you would need to be able to figure out how, how to track that employees capabilities and how they, they impact, uh, what they're doing on a production line. These are all types of new types of data, right? That you would have to capture and find out how to bring into these models in a way that they could understand it and actually enhance them. Now, the big question there is, do you have the money and, and the time to add to that, and does that really give you enough to justify the additional cost? And I would say in many cases, if you can get 85% of the value or 80% out of the value out of something simple, you could maybe move on to something else. That's another big chunk rather than trying to get that last little bit out of the, that artificial intelligence model. Um, maybe, maybe there's something that's a bigger bang for the buck that you haven't even tried to look at yet that you could again go and do a very basic model with, uh, that you could get some value out of. That would be more than you trying to get that last 10, 10, 15% out of the existing model.
Speaker 2:All right. So it real seems like there are many ways to kind of gain insight into the different types of data, but how, like, how would you know how to take action on the results? Uh, do you like to have any suggestions on how to get the most outta the investment, uh, in the AI ML solutions?
Speaker 3:Yeah, this is, this is, this could probably be a long conversation. We'll, we'll try, try and bring that down to a little bit more palatable, uh, answer. So the biggest issue is getting information from raw data is one aspect, which is what really our AI and ML solutions are doing, but then getting people to actually use it is, is actually one of the hardest things in the process. And so if, if companies are using AI models, um, trying to integrate them into your existing processes, and this is the key point in the most simple way possible<laugh> is really the biggest challenge and, and the biggest way to overcome issues. So when, when you talk about, um, how do you get information to cause, uh, a positive action? Um, some, some examples of that if, if we have, uh, a forecast that an impending asset, uh, is, is going to go down something as simple as saying, okay, usually I'll take, you know, that flag for oh, potential asset downtime, and I will send an email or I'll, I'll notify my maintenance department in some way, shape or form, but how, how do you just instantly, if you will include on the operat plan or the operational schedule show some sort of mark on their existing operational schedule that says, oh, I've got asset downtime as a flag. So that those people that are working with that operational schedule and that production schedule can see, Hey, I've got a conflict. Now I need to be able to utilize that and, and work with my maintenance team and say, okay, how can we, how can we deal with this? Um, how do we, how do we help prevent that? Or in the case of, let's say, I've got a large asset that doesn't come out of production for a long period of time. Let's say I've got a production window that is coming up. And it says, I'm going to do a bunch of maintenance, but this particular asset, I'm not going to in three months, but let's say six months from now, my prediction is saying that machine is likely to fail six months from now. My, my operational people can then contact the maintenance team and say, Hey, I see this flag out here. Maybe we should move up some maintenance in here and do that maintenance even at, even as the other sensors on the machine are saying, it's okay, let's fix it. It's, it's just making a simple change to the operations production scale that allows them to make use of that in a very, very simple way. I would say, you know, having automation, um, the more you can automate these results within a process. Uh, another good example, if you look at that is let's say my artificial intelligence, uh, is telling me that I have a key resource that might be at risk. How do I flag that to leadership with either an email, uh, or some sort of process that tells them, Hey, here's, what's going on this. We might be at risk with this employee, and here's the specific rationale behind it. Again, it's information delivered to them in a very simplistic way, but then they can take action for it. And that's frankly, I think that's one of the biggest problems that we have is many of these AI ML models produce numbers, right? And, and human beings don't necessarily work really well with numbers, if you will. They wanna say, okay, you know, I need to do something about this employee, right? It, it really, you're not gonna do something great with a number in a, in this human resource scenario. Um, you wanna know what's going on and why, and, and if you can translate the numbers into simple things, the human beings can deal with. I think you're gonna have a lot better success. And the other side of that is if you break down these silos of information, right? So all of these different things and who should consume that information, you start to drive, uh, a culture where you're no longer breaking down, or you're no longer having silos of information. You're breaking those silos down. Other people can see what's coming. And, and so now you start to develop what I'd call an insight culture, um, that, that there's a level of trust that comes out of that. Um, if you can kind of add some of that subtle nuance of simplicity, um, with, with still making the AI effective, uh, I, I think, um, just, you don't have to expose everybody to every little bit and bite of what the AI is saying. Just give them what they need and they'll able to do their jobs better with it. I think that's probably the, the, the best, uh, aspect of that.
Speaker 2:Okay. So you made it pretty clear, uh, that there must be like really clear integration and, uh, translation from numbers to actions. Mm-hmm<affirmative>. So now really a big question is how do you overcome that human element that's spoke about? And people's kind of resistance to change?
Speaker 3:You know, it's, it's interesting because I've had some differing opinions with leaders of companies that I've worked with on this, about it. The one thing we all agree on is leadership of these companies need to make it very clear, not only through words, but by their actions, that what we're working on is important. We need to support it. We need to adopt it even. So even though sometimes it might be painful and be open with the information required. I can tell you the death nail of any of these projects is when leadership puts it out there, they throw it at somebody, but they don't really send the right messages behind it, say how important it is, and really explain how important it is for everyone in the company. Here's the part that we tend to disagree though. And, and I, I'm more of a believer in how human beings behave than, than perhaps some leaders are. And that's this consideration about what's in it for me. Um, I, I believe that people tend to do things for their self interest<laugh> and I've actually had leaders of companies say, well, that's not our culture here. You know, we're all here all for one, one for all right. But I think that human nature is such at people that are working with these new types of tools. They really wanna know, all right, how does this make my job better? How does this make my job easier? How does it make my job more productive and me more valuable? And, and the one that, of course, nobody wants to talk about is, is this going cost me my job? And so leadership has got to have a very clear message about, Hey, if we can get rid of maybe some of these tasks that take up a lot of time, but aren't meaningful with AI and ML, um, we can provide other means of those employees to add new value to the company. And I think that's a little bit of a hidden element of change management, because I think people are afraid, Hey, I wanna, I wanna still have my job. I don't want, you know, the computers to take over, et cetera. And I, I still think, and I believe that this is well understood by many people in manufacturing, that we are a long way away from completely dark facilities in many industries. I'm not saying that there aren't those, those paths out there, um, particularly high tech manufacturing, um, and, and things like making computer chips and things like that, um, that that's a different realm if you will, where they can afford to, you know, spend tons of money to automate everything and put AI doing all of these different things. But there's a lot of manufacturing out there that I don't think lends itself to removing the human element, but if we can make that human element much better and have that consideration for them, I think you'll see a lot better adoption and, and, and change management along the way. I do think if you can define the goals at the outset that are both for the company and for the employee, and, and importantly develop a clear way of measuring that success and how are we doing toward those goals, then you're gonna have a much, much better, uh, where the workforce is gonna get behind the initiatives, uh, and leadership is gonna get behind the initiatives, but that, that defining success and how to measure it is really, really critical. Um, we, we, I I've, I've been part of projects where we have literally gamified, um, kind of the results of how people utilize the systems. And it's really interesting thing, uh, when people are invested, um, I even have, my, my nephew has, has, has worked on, uh, a project, uh, where I asked him question. I said, well, when, when you saw this thing come out and, and they posted the results up, um, of how we gamified the system, how did it make you react? And he said, oh, I sure as heck didn't wanna be the guy at the bottom, cuz I wanna win<laugh>. I think people really do want to win and, and how you measure that win, um, is just as important as showing those results and getting the buy-in. So the more, I think folks can maybe gamify, but do it in a way that is a win for everyone. I think that's the, the great way to kind of deal with some of, of the change management process. It takes a lot of effort. I, I think that change management and this measurement is actually the hardest part of any of these projects to get that right, I think is where the, the level of focus could be. But that's where you can, you can really rely on the knowledge of, I think plant management and some of the, the human resource specialists who really understand the culture of the company and what will work. So I, I think, um, that that can really, um, make a huge difference in the overall success of these projects.
Speaker 2:That's a really great point. Uh, do you have any type of closing thoughts for fit the shop?
Speaker 3:Sure. I think if, if you look at it, the act of utilizing and determining kind of what artificial intelligence or machine learning, uh, that you, you might want to, uh, go down, it requires some focus and skill sets that I think many companies, especially smaller, uh, companies do not currently have internally, I think seeking help from companies or individuals who have that type of experience such as Panorama. Um, I think it will really help clients get more quickly to a place where they can, they can describe a solution that would bring value to them and actually deliver upon that value. Um, it's if you're trying to start this from scratch, I will say is, uh, and, and I can remember back in the days when I first started working in these types of projects, we made so many mistakes, um, and, and just about cleaning data and how to prepare data and how and what the results really meant and how to get them into a format that somebody could actually use. It takes time. And you're trying to develop expertise, getting a shortcut by getting some help, seeking some help, um, is probably one of the best things you can do to cut down on that curve. But the other part is, and I think the general gist of what we've been talking about today is keeping it simple because simple actually leads to value more quickly than trying to start out with something complex. So those, those are the things I think the folks should think about as they're going forward. Um, you know, it, it, it takes a little experience to do it once you get on that path. I think you can, you can assume, you know, some of the roles, uh, and responsibilities for continuing down it, but, um, I, I certainly would recommend, uh, utilizing some experts who have worked in the space.
Speaker 2:Well, thanks for those, uh, final thoughts there. So thank you. I just wanna thank you very much for joining me the today, Paul, uh, discussion's really been food for thought, very eye opening. Uh, so I definitely appreciate your time.
Speaker 3:Well, I appreciate you having me here. Thank you.
Speaker 2:So if you'd like to inquire about Panorama's comprehensive services, feel free to visit our website@panoramaconsulting.com or you can reach out directly to our director of innovation, Alan Salton at Allen, do Alton Panorama consulting.com. Thanks again, everyone, and have a great day.